By Jayne Williamson-Lee
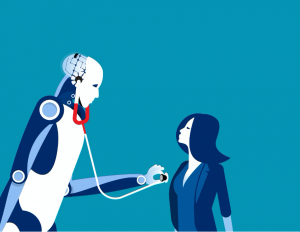
Problems of bias shake clinicians’ trust in healthcare algorithms, yet it hasn’t been agreed on just what trustworthy AI will look like. (iStock.)
Adamson, knowing that his patient was already at a high risk of this potentially fatal disease, went to do a biopsy. The sample came back from the screening: an early melanoma indeed. His colleague, an AI algorithm, had been wrong.
“The more I have used AI in my clinic, the less confident I am about how much more beneficial it is than current practice,” Adamson said in an interview. “Having a machine as another decision maker is fraught with uncertainty.” On a Feb. 8 panel at the American Association for the Advancement of Science annual meeting, Adamson and other experts discussed issues of trust and bias in artificial intelligence tools that are now being consulted in medical decision-making, such as in detecting cancer early on and predicting other health risks.
In the U.S., Black Americans have a much higher risk of cardiovascular disease than Caucasians. One predictive algorithm—the Framingham Risk Score (FRS)—didn’t get the memo, though, ruling that Black Americans’ risk was 20 percent lower than it actually is. Dr. Ravi Parikh, an oncologist at the University of Pennsylvania, discussed at the session why algorithms like FRS can be off in their predictions due to problems of statistical bias: U.S. minority groups including Black, Hispanic, and Asian Americans are undersampled, their data showing up less often in algorithms’ training datasets as a result.
In FRS’s case, the algorithm was fed data on what Parikh described as “overwhelmingly white” populations. “Having a one-size-fits-all algorithm that’s based on a primarily white population to dictate a lot of preventive care for Black Americans ends up being a problem,” Parikh said in an interview.
Existing social bias can also be baked into these algorithms, such as if models are based on data from primary care visits. Racial disparities affect whether some minorities can make it in for a doctor’s visit. To get a comorbidity code, for instance, you have to first be afforded access to healthcare. Parikh said, “Even not having that measurement can put an algorithm at risk for bias.”
Problems of bias shake clinicians’ trust in healthcare algorithms, yet it hasn’t been agreed on just what trustworthy AI will look like. As it stands, there is no consensus about what constitutes trust in AI or how to measure it. The panel speakers put forth ideas to guard against bias, such as to standardize datasets to ensure that Black Americans and other protected groups have adequate representation, and to label image data based on a gold standard to prevent faulty diagnoses from being automated. “The better the data, the better the model,” said Elham Tabassi, Chief of Staff in the Information Technology Laboratory at the National Institute of Standards and Technology (NIST).
National policy documents have laid out principles of trustworthy AI, stating that these tools be accurate, unbiased, secure, and able to explain their verdicts. The challenge now, Tabassi said, is translating these broader aspirational principles into technical requirements for AI that will make each tool’s trustworthiness measurable, an effort now being undertaken by researchers at NIST. “Standardized is our middle name,” she said about NIST at the session.
Encoding more trust in healthcare algorithms will help us to reach more patients. Vinton Cerf, Chief Internet Evangelist at Google and a co-organizer of and respondent at the session, noted in an interview afterward that “it’s hard to create human experts.” Compared to how many years it takes to train doctors in medical school and residency, AI are fast learners. Using computing, which is “infinitely expandable,” we have the possibility to “create a larger number of diagnosticians than we have humans available.”
AI tools may learn much faster than doctors—but not smarter. Adamson raised concern over image-based algorithms that diagnose cancer. These systems rely still on the consensus of pathologists—an external standard which is flawed, as pathologists can’t even agree among themselves in some cases on what constitutes cancer. “The gold standard for what is and isn’t cancer is up for debate,” Adamson said. AI will not resolve this uncertainty, only reproduce it. “It’s not getting you any closer to the truth,” he said.
Cerf, who posed questions to the panel about ways to expose flaws in existing paradigms, stressed that testing these applications against real-world scenarios is a large part of doing your due diligence as a computer scientist. “Machines don’t see like us,” he said. “They’re purely statistical and can get things wrong. At the very least, you want to be challenging these systems.”
Jayne Williamson-Lee is a technology writer and graduate student at Johns Hopkins. Her work has appeared in Psychology Today, OneZero, Behind the Code, and others. Follow her on Twitter @jaynew_l or email her at jwilliamsonlee@gmail.com.
This story was edited by NASW member Elliot Richman, who served as Williamson-Lee's mentor during the NASW-AAAS Spring Virtual Mentoring Program.